Conformalized Interactive Imitation Learning: Handling Expert Shift and Intermittent Feedback
Michelle Zhao, Reid Simmons, Henny Admoni, Aaditya Ramdas*, Andrea Bajcsy*ICLR, 2025
project site / paper / bibtex
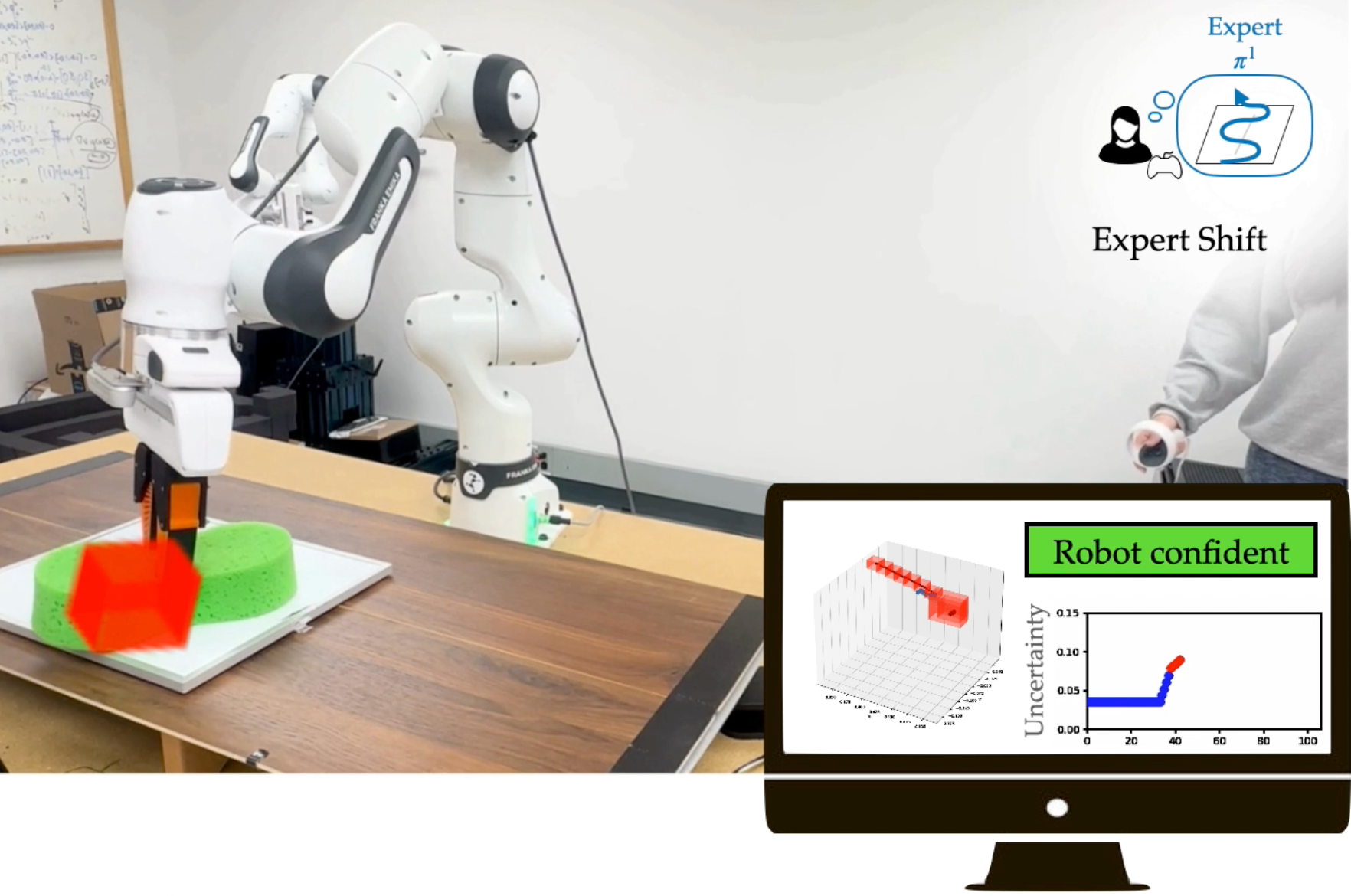
Uncertainty quantification offers a way for the learner (i.e. robot) to contend with distribution shifts encountered during deployment by actively seeking additional feedback from an expert (i.e. human) online. From the conformal prediction side, we introduce a novel uncertainty quantification algorithm called intermittent quantile tracking (IQT) that leverages a probabilistic model of intermittent labels, maintains asymptotic coverage guarantees, and empirically achieves desired coverage levels. From the interactive IL side, we develop ConformalDAgger, a new approach wherein the robot uses prediction intervals calibrated by IQT as a reliable measure of deployment-time uncertainty to actively query for more expert feedback.